14 CEOs Break Down the Challenges in Quantum
Insights from GTC 2025!
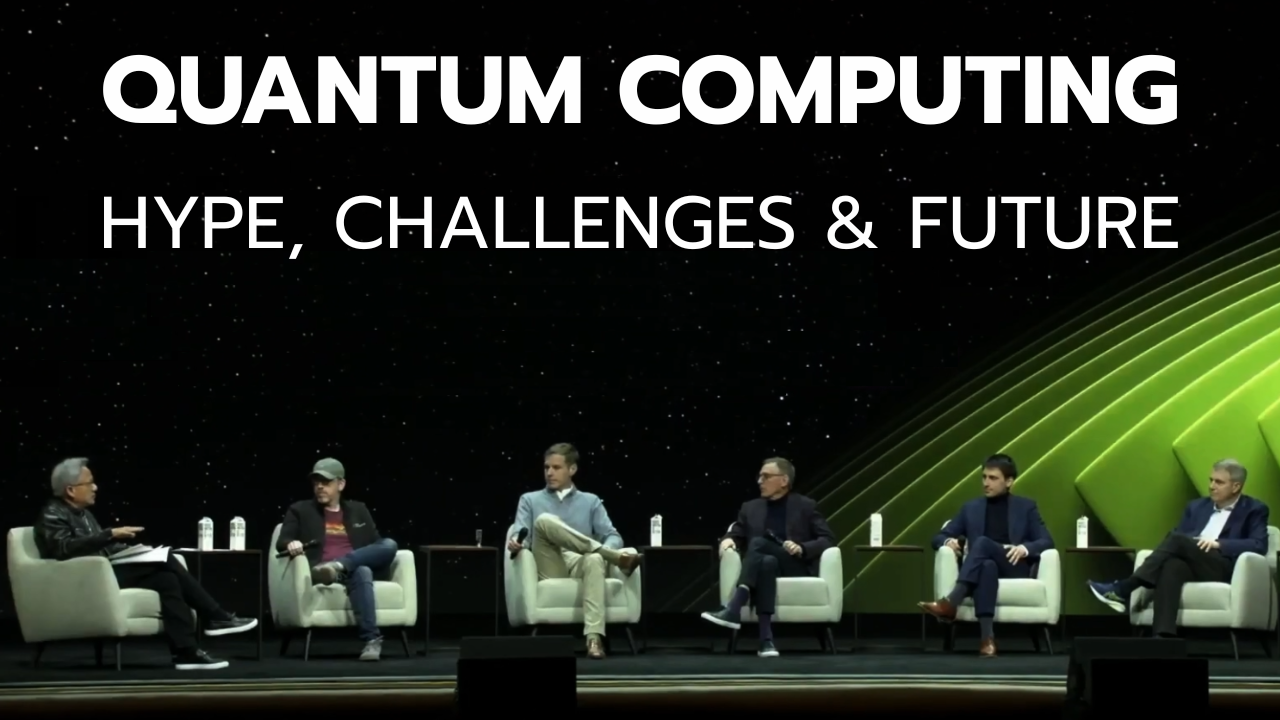
Watch the video!
I attended Jensen’s Quantum panel with 14 leaders in the field at GTC, and it was the “coolest” one.
Pun intended with “coolest” (quantum computers literally need to be cooled near absolute zero!).
With all my time spent in AI, quantum has always felt a bit abstract and hyped. Yep, even for someone in “AI” (with everything from generative AI to agentic AI, and whatever in between), Quantum feels even more hyped without actually delivering. As Jensen said it so well, there’s almost a 1:1 breakthrough to controversy ratio in that field. Just like Majorana 1’s recent Microsoft “huge breakthrough,” — where are the actual results and industry use? Why do we see all this hype but no actual follow-ups?
The promises are that quantum computing could fundamentally change what’s possible in science and technology. The real excitement around quantum is its potential to tackle problems that are simply impossible for today’s most powerful supercomputers — like accurately simulating complex molecules, materials, and biological processes. This could lead to breakthroughs in developing new medicines, creating better materials for batteries, or finding more efficient ways to generate clean energy. It could even help us understand fundamental physics more deeply, revealing insights about the universe at its smallest scales. Quantum computers might also accelerate progress in AI, by generating highly precise data to train models or solving optimization tasks that currently take massive computing resources.
So, despite all these exciting promises, quantum computing often seems shrouded in hype and confusion. This panel however actually helped me cut through some of that noise and so I wanted to share some bits from it.
Now, before we get to it, to be clear, I still don’t completely understand everything happening in the quantum space and would love inputs from any experts on these, but here are some valuable insights I took away after the panel and with some more research on my own…
My biggest takeaway? Quantum computing isn’t about replacing your computers anytime soon — it’s about specialized hardware (QPUs) tackling specific tasks alongside classical processors. Just like GPUs are added to computers as an additional chip along with CPUs, QPUs (“quantum processors”) could be a third option for some very specific uses cases. Just like we use GPUs for gaming and AI.
The challenge is that even quantum computing experts aren’t yet certain about the best practical use cases for quantum processors — or “QPUs.” But there’s an even bigger obstacle: actually building reliable quantum hardware. And when it comes to making these chips usable, there are two major problems…
First, what we call the quantum “error correction”:
Quantum chips regularly produce calculation mistakes (“errors”) because qubits — the fundamental units of quantum information that can represent multiple states simultaneously — are extremely fragile. They easily lose their delicate quantum properties (a process called decoherence) when exposed to tiny environmental disturbances like heat, vibrations, or electromagnetic interference. Without robust error correction techniques, quantum computations quickly become unreliable. To solve this, quantum computing relies on the concept of logical qubits — abstract units of quantum information encoded across many physical qubits. Experts estimate that it currently takes about 100 physical qubits working together to reliably represent just one logical qubit, dramatically increasing the complexity and size of quantum computers. This enormous overhead highlights just how challenging it is to scale quantum systems while maintaining accuracy and precise control.
The second problem is scalability:
Today’s largest quantum computers still have only dozens to a few hundred qubits — far short of the thousands or even millions needed to tackle real-world problems like simulating complex molecules or optimizing large-scale logistics. Adding more qubits isn’t as straightforward as adding transistors to a classical chip: every qubit must be precisely controlled, individually wired, and carefully shielded from interference. As the system grows, complexity skyrockets. For example, in certain superconducting quantum processors, each individual qubit currently requires multiple dedicated wires — sometimes up to five per qubit — to provide precise control signals. At that rate, a quantum system with a million qubits would demand millions of wires, creating massive engineering and reliability challenges.
And what about the solutions to these two problems from these 14 CEOs of leading companies?
“We don’t know”
Yep, there’s no one clear path forward. They are still exploring how to tackle those problems but also for what Quamtum processors could actually be useful.
From the 14 companies in the panel, some of them were trying entirely different methods for stacking Qubits, mitigating these errors and making the global system work…
One approach uses superconducting circuits, which require being cooled down to temperatures close to absolute zero — just milliKelvins above. At these incredibly cold temperatures, superconducting circuits can tap into quantum properties, making techniques like quantum annealing possible. This method is especially good at solving optimization problems, like finding the most efficient routes or solutions from countless possibilities. However, superconducting systems aren’t very flexible for general-purpose computing tasks and face significant hurdles around noise, errors, and scalability. Companies like D-Wave and Rigetti are prominent examples of those using this approach.
Another completely different method involves trapped ions manipulated by lasers. Here, individual charged atoms — ions — are held in place with electromagnetic fields inside ultra-high vacuum chambers. Lasers then precisely control and interact with these ions to perform quantum calculations. A big advantage of this approach is that it operates at or near room temperature and achieves very high accuracy and fidelity. The main challenge, though, is scaling: as the number of ions grows, controlling them accurately becomes increasingly complex. IonQ and Quantinuum are two leading companies working on this technology.
There’s also a promising third path: neutral atoms controlled by lasers. Neutral atoms are held in optical traps and carefully arranged using laser beams, creating dynamic qubit structures. This approach, which operates around room temperature thanks to laser cooling techniques, has exciting potential when it comes to scalability — potentially handling thousands or even more qubits. However, it’s still relatively early days for neutral-atom quantum computing, with companies like Pasqal and QuEra actively pioneering developments in this area.
Finally, a very different — and still theoretical — approach is known as topological quantum computing. This method aims to overcome the noise and error problems faced by other approaches by using exotic particles called Majorana fermions. Unlike conventional qubits, topological qubits store information in a way that’s inherently protected against errors, almost like tying a knot in a rope — no matter how much you move or shake it, the knot remains intact. This built-in protection could significantly simplify error correction and improve reliability. However, there’s a catch: no one has yet conclusively demonstrated a functioning topological qubit in practice. Microsoft is one of the major players actively researching this promising yet challenging approach.
And there are other approaches. In short, multiple approaches are advancing simultaneously, each tackling quantum computing’s challenges from different angles. And there’s no consensus on where to go next, for now. But they all expect to have one of the directions to come on top of the others as we advance one discovery at a time, just like how, in the mid-1990s, the graphics industry debated between two approaches: quadratic texture mapping and triangle-based rendering. Nvidia’s first product, the NV1, utilized quadratic texture mapping, aligning with Sega’s approach. However, it seemed to be the wrong choice, and it almost went out of business. As industry standards and research evolved, triangle-based rendering clearly became dominant, leading Nvidia to shift technologies for all following products.
What about Nvidia here? Interestingly, Nvidia’s role isn’t to build quantum computers directly. Instead, they’re creating a research center in Boston to help integrate quantum processors with AI-driven classical supercomputers. Think GPUs and CPUs doing the heavy lifting while QPUs handle specialized quantum tasks.
So, what are these “tasks” that quantum systems could tackle? As I said, even the experts don’t know yet. They gave examples of using QPUs for generating labelled data for training AI systems, mostly for biology, physics, or material science.
After researching a bit, I got that quantum processors can natively simulate complex quantum systems — capturing effects like superposition and entanglement that we often hear — which classical GPUs can only approximate with significant simplifications. This means QPUs can generate higher-fidelity, more accurate labeled data, especially for tasks in quantum chemistry, biology, and materials science, where true quantum behavior is essential.
And quantum behavior here refers to the unique ways particles act according to the laws of quantum mechanics. For example, superposition allows a qubit to exist in a combination of 0 and 1 simultaneously, and entanglement links the state of one qubit to another, regardless of distance. These behaviors produce quantum effects — measurable outcomes such as precise energy levels of electrons, their probability distributions, and interference patterns in a molecule. In other words, when a quantum processor simulates a system, it directly captures these intrinsic quantum interactions rather than relying on classical approximations. This results in high-fidelity data — accurate labels like energy states or reaction probabilities — that can be used to train AI models for applications in chemistry, biology, and material science.
If you didn’t get that completely, don’t worry. It is honestly still a bit unclear and abstract to me too, even after a few deep researches, if anyone can help clarify that?
One of the companies, Infleqtion, took a super interesting approach to the problem, trying to first commercialize simpler quantum technologies — like highly precise quantum-based clocks and sensors — to validate their tech. Their strategy is to gradually refine the core technology through practical, lower-risk products before scaling up to more complex quantum processors. This helps them bridge the gap between quantum theory and real-world usefulness, and might point to how quantum companies can find their footing even before the “ultimate” quantum use cases become clear. Again, mainly to improve upon what exists and not replace computers. This is just like what Nvidia did with first tackling the video game industry where the stakes were lower (nobody minded a few dropped frames or missing pixels in a game), which allowed them to gradually scale their GPUs into powerful tools used widely today, from AI and autonomous vehicles to scientific research, all by mainly adding benefits to existing technologies with no downsides. Quantum computing might similarly need to find a practical “stepping stone” industry to grow and mature.
So, despite all the buzz, quantum computing today feels like where AI was a decade ago: lots of potential, tons of uncertainty, and a big open question (or more) about what applications will truly matter.
This isn’t surprising, though — every major technology, from the internet to electricity, faced the same skepticism at first. Even classical computers initially had unclear use cases; early on, they were mainly employed for specialized military applications, like precisely calculating missile trajectories during wartime, long before becoming the general-purpose devices we use today. It always takes time to understand these new paradigms and uncover the practical applications where new tech can truly shine. Quantum computing will likely follow a similar path, gradually transitioning from specialized tasks to broader uses.
I’m starting to get a bit more interested in Quantum. Not for its hype but simply for the complexity of the task and to better understand how it works.
And what about you? Are you bullish or skeptical about quantum computing’s near-term impact? I’m curious to hear your thoughts.