AI Deep Learning Explained
Deep learning with a simple analogy
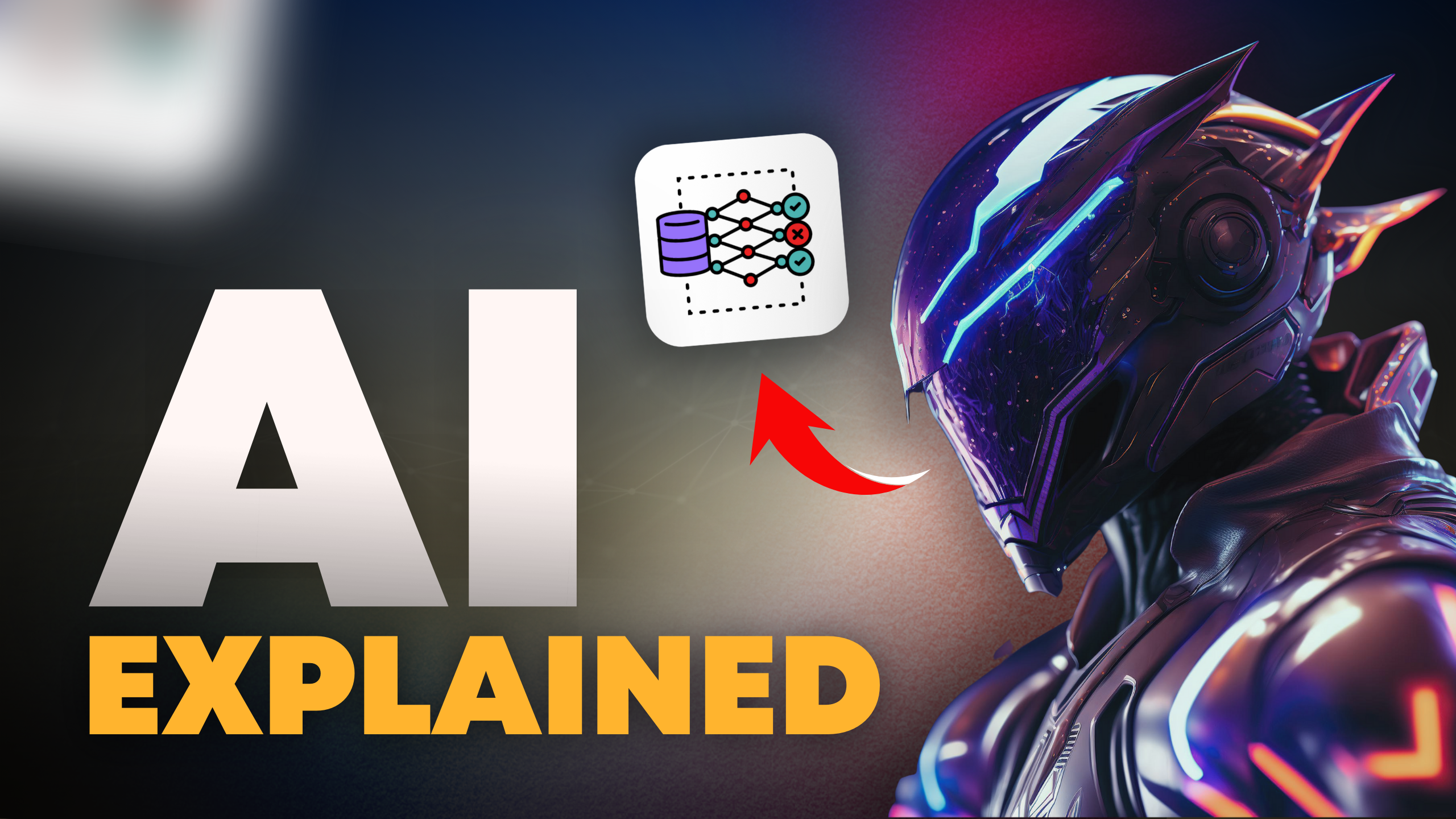
Watch the video...
When we talk about artificial intelligence, or AI, we tend to mean deep learning. Summarising text, generating sentences, removing the background of images, recommending the next movie you should watch, or even detecting cancer earlier, deep learning powers much of the state-of-the-art technology in the world right now, including ChatGPT or MidJourney. Although useful and increasingly powerful, is this intelligence? Let’s begin by imagining a group of computer scientists and a very large elephant in the same room. Nervous grins and darting eyes follow whenever someone points at the elephant and asks a question about AI and intelligence. It seems like our gut knows what intelligence is, but our tongue struggles to put it into words. We seem to always change our minds about intelligence. So rather than focusing on answering if deep learning is intelligent or not, let’s be proper computer scientists and focus on what it can do and how. Anyways, who cares if it is really intelligent if it can drive perfectly for you or digest long emails and answer for you?
A Deep Learning Analogy...
You can see AI, or deep learning, as a bad student.
It will go to an exam without understanding the matter covered. Instead of studying beforehand and learning the materials from the book, it will try to hack its way to the passing grade.
Like a bad student desperate for a pass, it will try to copy its peers without understanding the questions themselves. The only difference is that it’s a bit cleverer than most bad students in one remarkable way: it won’t get caught easily and will look like it studied.
Instead of copying one or two students next to it and getting caught by the professor, it will take the answers of all students in the class and copy the most recurring answer for each question. So it will still only copy and paste answers, but a bit more intelligently, using statistics. It will understand what the most likely answer to be given to any question using everyone’s copy is. This process will be repeated for all exam questions. So you might have the best grade in the class, but you still won’t be understanding the matter covered, and you won’t get caught cheating since all your answers make sense as they are based on other peoples’ answers and not just one person. If you scale this process to the whole internet and all topics in it, you get GPT. Then, if you copy even further real conversations from humans and practice with them, you get ChatGPT.
This is how AI works. It is basically a ‘fake it till you make it’ machine. It looks impressive, but it doesn’t understand what you want it to achieve. It just looks like it does. By feeding it a lot of such examples like a question and the students’ answers, it will learn the optimal solution to pick for similar questions. This is if the students are good enough, and it is also why your data is so important! If you feed it enough examples from experts, or in this case, from great students that really studied, it will be powerful, like ChatGPT, compared to previous models that you probably didn’t even hear about!
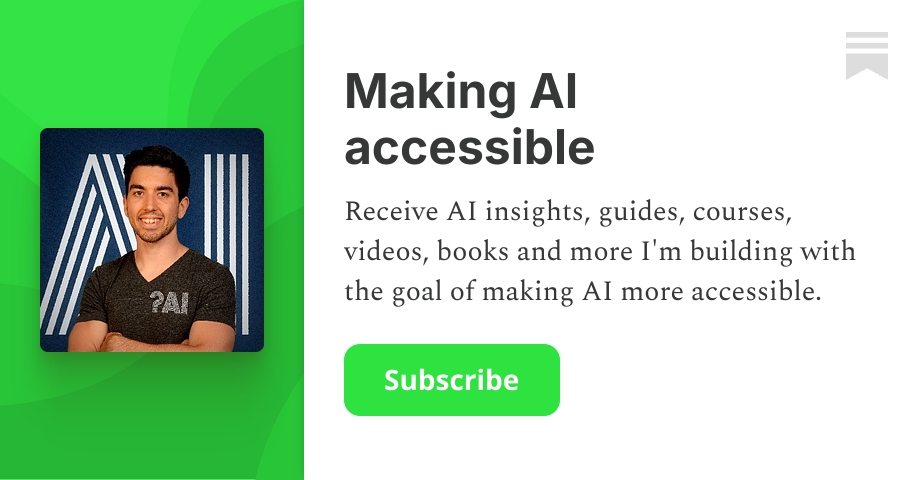
At this point, you may be wondering, how does deep learning fake it? The details are a topic of another article, but it may be useful to understand what a loss function is. The loss function is the driving force behind deep learning. Imagine a teacher telling you how many points you are missing from a full mark. It’s impossible to know an exact score, but a good loss function will give you an idea of how well you are performing. The trick here is that the teacher grading your exam does not tell you which questions you failed precisely. They don’t even give you any feedback besides an approximate score of how much you failed. Thus, it’s up to you to figure out what you did wrong and how you can get better. This is why it takes a lot of data and trials to train a powerful deep learning model.
AI - Weak and Powerful at the Same Time
This is why AIs are both powerful and weak.
They are powerful because they will have excellent results if you have the data to teach them what you want.
They are weak because they won’t be intelligent at all outside of the data they saw. It won’t learn the matter covered for the exam, but what the students are likely to answer. So your AI won’t understand maths or history. It won’t understand what a tumor is or what your boss wants from you. It will just understand statistics very well for the current exam and replicate what most doctors would do or what most bosses want from someone like you. Such an AI trained for a History class exam won’t be able to summarize the class, help someone, or do anything other than answer this particular exam or very similar ones.
This is how AIs can generate text, edit images, understand trends, detect cancer earlier, or recommend the next movie you should watch. They are all different, yet very similar, AIs trained only to achieve such a task using examples. An important step that most AIs do during their training is called supervised learning, where AIs are told what to find or do using examples produced by experts. We call that supervised because they follow experts and how they make a decision but do not (and will never) have their knowledge this way. They are under expert supervision and can only do as well as they at best.
An AI isn’t trained to understand what a brain is or what a tumor is. It is trained to answer what the majority of neurologists would answer seeing the same brain image. Here I want to stress the part where I say the image must be “the same”. Indeed, you might imagine that medical imaging from different hospitals can use the same AI, but this might not be the case. For instance, most hospitals have their own settings for MRI machines that take pictures of your brain or spinal cord. This causes the AI to not even recognize the image and what is displayed due to contrast variations which we wouldn't mind. Our poor student is at a complete loss: it’s like the questions of the exam were reworded and reordered, and now it cannot rely on what it learned. In contrast, an expert neurologist might need a bit more time to look but will quite easily still see what’s wrong in an MRI scan from another hospital.
And if this issue sounds interesting, you’ll love my upcoming videos on AI and the biomedical field, as this is my Ph.D. thesis, and I will share a small series of videos about what I am doing with AI and MRI images!
Conclusion
This is the current state of Artificial Intelligence, and more precisely, deep learning. Unlike humans, deep learning, in many ways, learns answers, while humans tend to aim to learn about the topic at hand. This reason is why deep learning is powerful for narrow use cases when you have available data. In other words, this difference is due to it learning to succeed in such a task rather than learning the knowledge required for the task.
I may have underestimated this bad student a little… And I want to apologize publicly, especially with what we’ve seen with ChatGPT recently. It may be lazy, but sure knows statistics well, which is why it can be used for so many different kinds of applications. And going through the answers of everyone in your exam hall cannot be easy either. There are a lot of engineering prowesses and tons of incredibly innovative learning techniques researchers developed and keep developing to improve them. But what if you actually tried to understand the material? This is what symbolic AI attempts to do, but it’s a topic for another article!
I think deep learning doesn’t equal intelligence, and it doesn’t really matter if it does or not, but it’s still pretty interesting to discuss what kind of intelligence it has and what is its understanding of our world. I’d also love to know what you think in the comments below. Currently, AI isn’t quite like us. In another article, we’ll dive deeper into the differences between AI and humans, which you should subscribe and stay tuned for. Even though it is different, it does not prevent the fact that artificial intelligence models are very powerful and valuable, nor that this is the final state of AI. A bad student has other qualities we can leverage! Qualities that I have already explored in hundreds of articles!
Remember that what I described here is simply an analogy for how Deep Learning works, and mostly one of the learning techniques called supervised learning, the most common form of artificial intelligence in today’s applications. Researchers are continuously improving how it works, and what we call “AI” might not be the same in a few years, but only the future will tell—what are you hopeful or scared about when it comes to deep learning? I might select your answer for a future article!