The Future of Video Generation: Deep Dive into Stable Video Diffusion
Stable Video Diffusion Explained
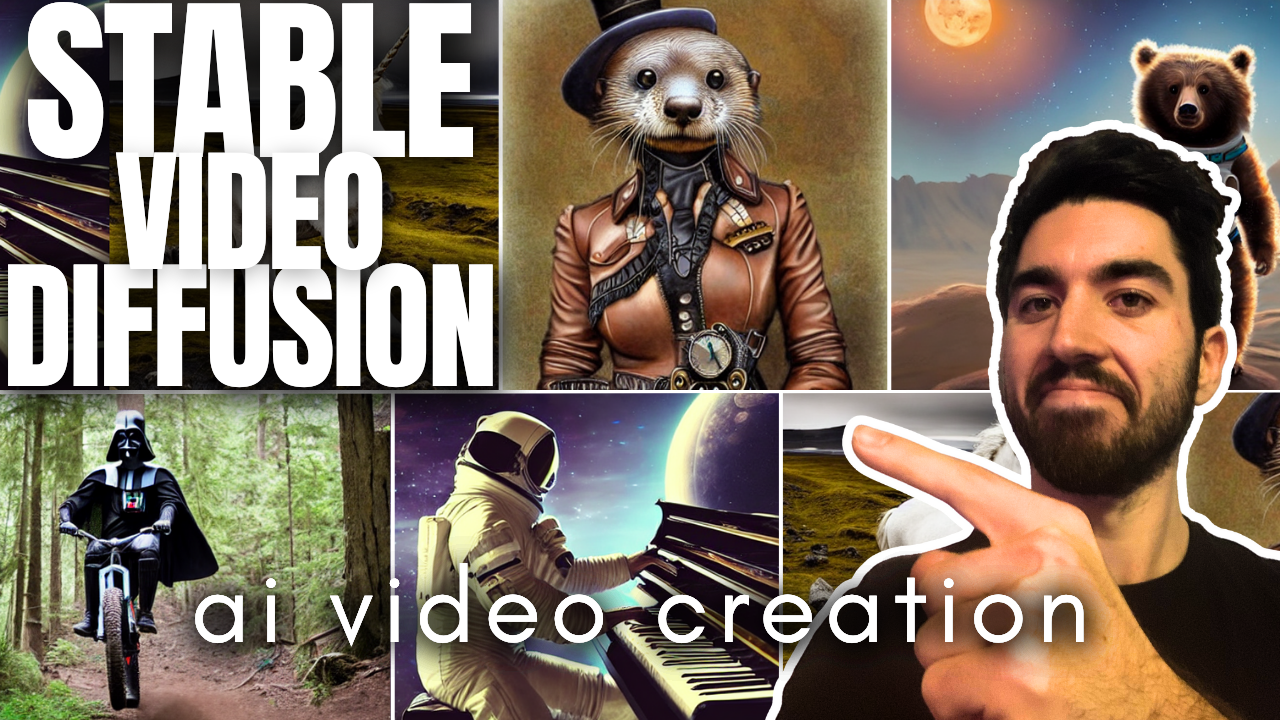
Receive my blogs and more on my AI newsletter and receive free gifts, such as my secrets to success on YouTube!
Watch the video
What do all recent super-powerful image generation models like DALLE, or Midjourney have in common? Other than their high computing costs, huge training time, and shared hype, they are all based on the same mechanism: diffusion.
Diffusion models are the state-of-the-art results for most image tasks, including text-to-image with DALLE but many other image generation-related tasks too, like image inpainting, style transfer, or image super-resolution.
Then, latent diffusion or the well-known stable diffusion came out, changing everything when it comes to image generation.
But I am not here to talk about old news. We are here to go over the new paper and model released by Stability AI: Stable Video Diffusion. The most recent and open-source video generation model that you can use right now! It takes either images or text and is able to generate cool videos like these automatically. It can even be used to generate multiple views of an object as if it were in 3D.
I’m Louis from What’s AI, and let’s dive into how this new model works!
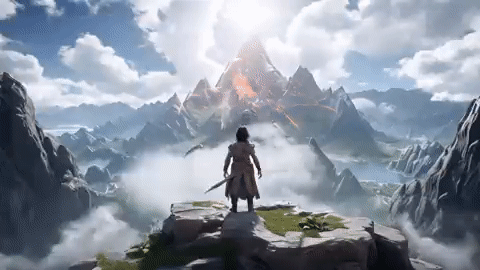
Before getting to videos, let’s do a recap on how Stable Diffusion works for images.
Stable Diffusion made training and processing images more efficient and accessible by operating in a compressed or latent space rather than directly on high-resolution images. This approach involves encoding an input (which can be text or an image) into a lower-dimensional representation. This basically means to teach a model to extract the most valuable information just as we would store a concept in our brain. If you see an image of a cat or see the word cat, it will both mean the same to you. It’s the same thing with the model’s encodings, where all information is placed in a space that makes sense for the model. By seeing thousands and thousands of images and text, it is able to build such a representation that is both compressed, information-efficient, and with high value compared to an image with lots and lots of pixels that aren’t really useful. See here where not even a third of the image is relevant, showing the cat.
The model then learns to generate images from this space using noise.
Imagine you have a blank canvas where you want to create a picture. In diffusion models, we start with this canvas not completely blank but filled with random scribbles and patterns, which we can call “noise.” This noise doesn’t have any meaningful pattern or structure — it’s just random.
The job of the diffusion model is like that of an artist who will gradually transform these random scribbles into a coherent, detailed image. It does this in a step-by-step process. At each step, it carefully adjusts the scribbles, slowly shaping them into recognizable parts of the image. This process is guided by the model’s training, where it has learned how to make these adjustments by looking at many examples. So we initially took many image examples and randomly applied noise to them, which you can see as scribbles, and provided those scribbles to the model. Then, we ask it to try and re-create the image. Since we know all the scribbles that we progressively gave to the model, we can correct it and make it learn to give the right scribbles at each step.

The underlying concept is that the model, during training, has learned the right parameters to apply to the noise to recreate an image that closely resembles the training images. And, as we saw, this is all done in a compressed space. The last thing we need to do is to go back to a real image instead of this model’s representation, which we call encoding. So we need to decode or transform this latent representation back into a high-resolution image. It’s basically like taking an older, damaged, or unclear small painting and re-drawing it on a very large canvas. It will be the same picture but much bigger. The decoding process involves reconstructing the detailed features, textures, and colors that were compressed in the latent space, effectively ‘upsampling’ the image to its full size and resolution.
We can now generate a new image efficiently, sending only some text as a prompt as we’ve all been playing with either using DALLE, MidJourney, or Stable-diffusion-based apps.
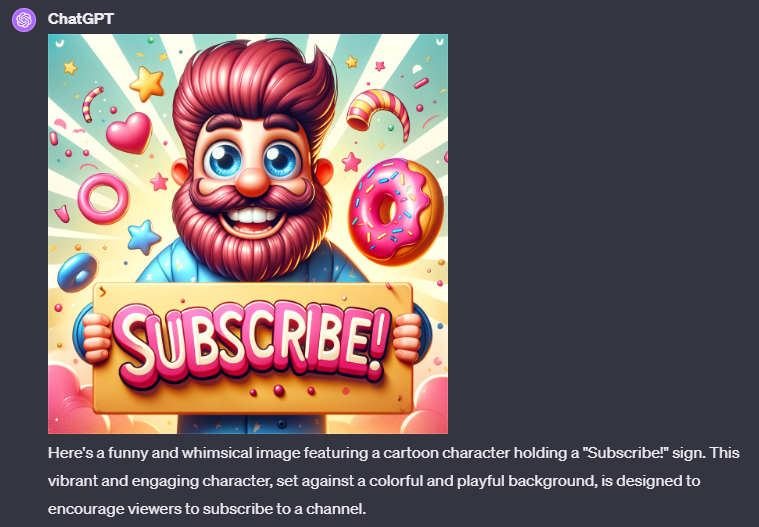
When moving to video, the challenge is not just to transform noise into a single image, but into a series of images (frames) that change over time in a smooth and consistent way. Consistency is the biggest issue here, as no errors are allowed for credibility. We can directly spot when something’s wrong since our brain is wired to spot anomalies in nature to survive. It just feels off and weird if something like this happens.
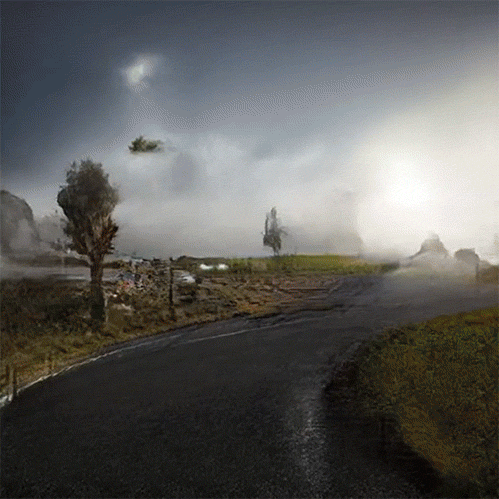
Dealing with time, or in other words, videos requires additional capabilities in the model to understand and replicate how objects and scenes change over time — essentially, how to adjust the noise not just for one image, but for a flowing sequence of images that make up a video.
Latent Video Diffusion Models:
Just like its image counterpart, Stable Video Diffusion operates in a latent space, but with a critical addition — it includes temporal layers. These layers are specifically designed to handle the dynamics of video sequences, focusing on the continuity and flow of frames over time. This is extremely important as we cannot simply generate 30 images and squash them to make a video. Each frame needs to have access to the others during training in order to ensure consistency.
Fine-Tuning on Video Datasets:
To achieve realistic video synthesis, Stable Video Diffusion is pre-trained with images using Stable diffusion and then re-train a second time with videos with this temporal layer added. The pre-training on images allows the model to understand our world with lots of examples of various objects and scenes. Then, the model learns to replicate complex aspects of videos, such as movement, changes in scenery, and interaction between objects, with a similar training process but with multiple frames generated at the same time. Finally, for quality, we add a final fine-tuning step where we repeat this video training process but use only high-quality videos to further improve the results.
Handling Motion and Change:
In videos, not only does the appearance of objects matter, but so does their movement and how they change over time. The object cannot change colors or shape over time. It also needs to follow real-world dynamics, depending on what it is. It moved differently, if it’s a rock or a cat. The model is trained to capture these dynamics, ensuring that the generated videos have a natural and fluid motion.
For the most technical of you, Stable Video Diffusion incorporates temporal convolution and attention layers. So the same approach as in most recent vision models is to produce more representations through feature maps and have them share information with each other. These layers are added after every spatial convolution and attention layer in the regular stable diffusion model, enabling the generation of consistent and coherent video sequences from text or image inputs.
Comprehensive Video Representation:
Stable Video Diffusion provides a robust video representation that can be fine-tuned for various applications. This includes not just text-to-video synthesis but also other tasks like multi-view synthesis, making it a versatile tool for different video generation needs.
The model achieves state-of-the-art results in multi-view synthesis while requiring significantly less computational resources than previous methods.
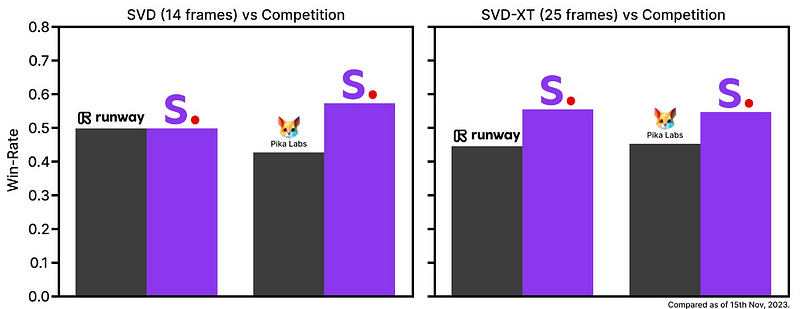
Just like stable diffusion for images, it makes video generation more accessible for a wider range of users and applications.
Of course, it’s not perfect, but it’s getting there. Long videos are still quite more challenging than short ones. They also found that their approach didn’t generate lots of motion in videos. If you try it, I’d love to know your thoughts on the results you get! In any case, sharing this new open-source model is a very good step in the right direction. I’m excited to play more with it and see what people build from this model.
I hope you’ve enjoyed this overview of the Stable Video Diffusion model, I invite you to read the full paper for a deeper understanding of the approach and their data curation process to build the datasets and further improve the results. They also shared lots of amazing insights to build and train better generative models.
If you want to stay up-to-date with these videos and the interviews I do, subscribe to my free newsletter, where I share all my projects and insights related to artificial intelligence.
Thank you for reading the whole article, and I will see you in the next one with more AI advancements!
References
- Code + some examples: https://github.com/Stability-AI/generative-models
- Blattmann et al., 2023: Stable Video Diffusion. https://static1.squarespace.com/static/6213c340453c3f502425776e/t/655ce779b9d47d342a93c890/1700587395994/stable_video_diffusion.pdf
- Examples and blog post: https://stability.ai/news/stable-video-diffusion-open-ai-video-model?utm_source=twitter&utm_medium=website&utm_campaign=blog
- Model weights and card: https://huggingface.co/stabilityai/stable-video-diffusion-img2vid-xt
- Stable diffusion video I referred to: https://youtu.be/RGBNdD3Wn-g