What is Explainability AI?
Interview with Yotam Azriel, CTO at TensorLeap - What's AI episode 13
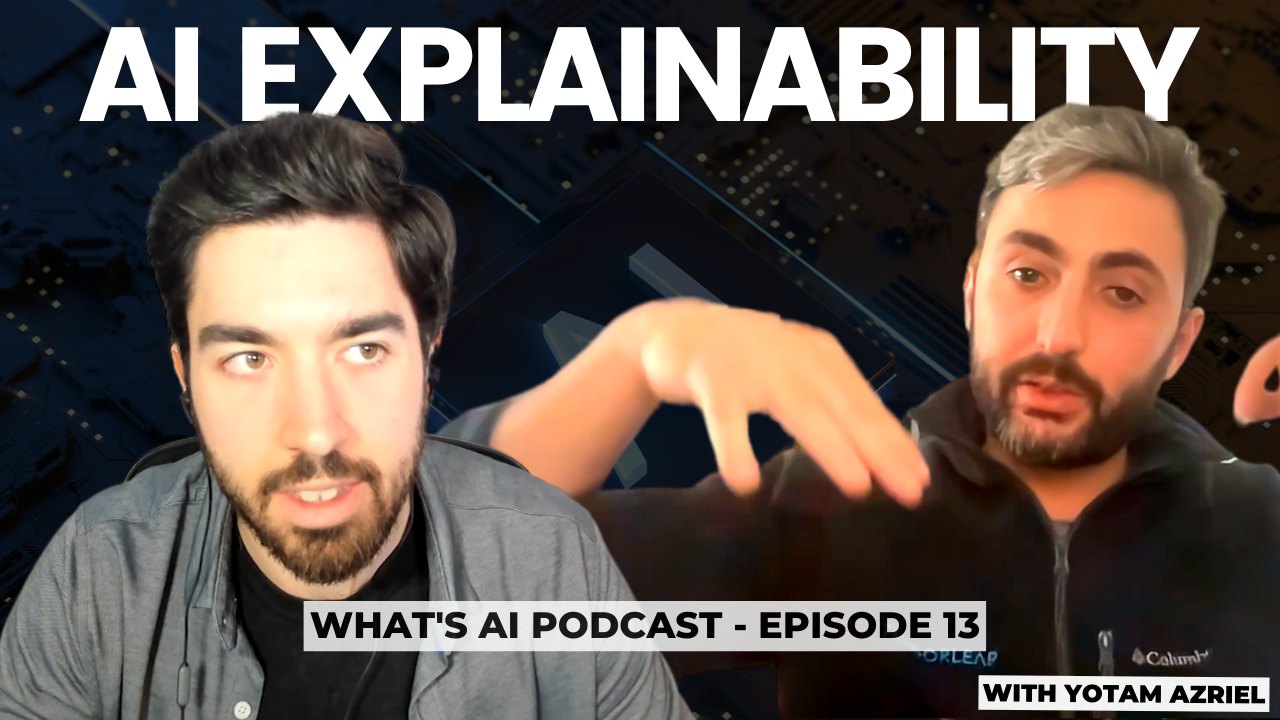
Join us this week for a fascinating conversation on the What’s AI podcast, where Yotam Azriel, co-founder of TensorLeap, shares his fascinating journey, insights, and vision for the future of Explainable AI. Discover what the power of passion, curiosity, and focus can accomplish! Yes, I didn’t mention school or university voluntarily, as Yotam is a successful data scientist… without any formal university degree!
Here are some insights from this week’s episode before you commit to a knowledge-packed hour-long discussion…
Yotam Azriel, despite not following a traditional academic path, embarked on a scientific adventure at a young age, exploring fascinating realms such as magnetic fields in physics, wireless charging technology, and AI. These diverse experiences shaped his knowledge and prepared him for his entrepreneurial endeavors.
What sets Yotam apart is his approach to learning: hands-on experience! By immersing himself in unfamiliar domains and clear expectations, goals, and deadlines, he acquires essential skills while staying firmly focused on his objectives. This method offers a relatable and effective alternative for self-learners like you (if you are reading this!).
We dive into the world of the AI startup TensorLeap — an applied explainability platform that empowers data scientists and developers working with AI models. By tackling the challenge of understanding complex AI behavior, TensorLeap is revolutionizing the landscape of explainable AI, an exciting new field with lots to discover.
Gain a deeper understanding of Explainable AI, where the goal is to clarify decision-making for users and utilize mathematical techniques to comprehend neural networks. TensorLeap focuses on the latter, providing valuable insights into AI systems.
Looking to enter the field of AI? Yotam Azriel’s advice is to pursue something applied and tangible! By setting clear goals with real-world outcomes, you’ll find the motivation needed to learn and thrive in the exciting world of artificial intelligence.
Don’t miss this captivating podcast episode with Yotam Azriel as our special guest, interviewed by me (Louis Bouchard) for the What’s AI podcast. Tune in on Apple Podcast, Spotify, or on YouTube and expand your knowledge of Explainable AI!
Here are the questions and timestamps to follow along or jump right to the question that interests you most…
00:26 Who are you and what’s your background?
04:39 And how did you find that job?
06:14 What would you recommend to someone from a different background who wants to get into the field?
08:23 So would you recommend having some sort of incentive to learn?
10:05 Why do you assume you’re not cut off for academia if you like writing and reading papers?
15:00 What is Tensorleap and what do you do there?
21:28 What is Explainability AI and please give an example of a technique?
27:35 Could you give an example of how an explainability AI technique could help improve or understand the SAM model?
31:39 Would the same explainability AI technique work for different architectures?
36:30 Can we do anything to understand the results of the model better when we work with pre-trained models?
40:20 What are the basics for someone new to the field who wants to get into explainability AI and use it to better understand their model and its results?
42:48 Should everyone creating AI models also be familiar with explainability and be able to understand the decisions of the models they create?
45:21 If the model’s explanation is right or not you might need an expert in the specific field?
52:20 Is there a way to better understand models that are used through API’s, their decisions and why they answer the way they did?
56:15 Is the ultimate goal for explainability AI to do anything it can to understand the AI model?
58:50 Did you search into neurosciences and try to implement some of their ideas on artificial networks and vice versa?
01:01:20 How do you see the explainability AI field in 5 years?
01:03:27 What are the possible risks with explainability AI?
01:04:56 Who should use Tensorleap and why?
Audio Transcript
This is an interview with Yotam Azriel, a friend of mine and co-founder of Tensor Leap, a company focused on explainability ai. And this is also the goal of this interview. We cover explainability ai, what it is, how it works, and how to do that. I hope you enjoy the interview. What is your academic background and your background outside of academia?
I have no academic background at all. I I started to work just out of nowhere. I, I, I did some research in some university in the staff. I started my scientific professional life very early. I started to work in IT companies since 16 to do some research in different places. So I started in, in physics.
Mostly department. I did some magnetic fields, infer magnetic materials, research how magnetic field is, is floating, how you can improve transformation of, of energy. And I worked on some patent on a, on a few researchers. I got, oh, reward from the Israeli president for one of my research when I was about 16.
And then some company found me, called me, and I joined them. It was Paloma. We did wireless charging technologies. I had a small venture back then. He erased some money, tried to work on some kind of neural transformer. And, and then the, I joined the Israeli army for, for intelligence. So I, I developed some techniques to detect stuff.
And, and from there I moved to the nuclei research and move to the nuclear research Center, which is. A research center in Israel. And and I think that, that it's, I had one conversation with one crazy physicist that came to me and told me I was sure that I, I wanted to be huge physics. I, I thought that about Bar and Einstein and Schneider.
And then this guy came to me and told Newan, there is no, and there will never be again, any big physicists. Those people are. And that it's, it's thousands of people that working on a very complex and and a very comprehensive theory and everyone changing very small piece in this huge theory. So you won't be a physicist, but by the way, this field of biological algorithms that can learn seems to be a serious thing and you should go there.
So, so I started to focus mainly on AI back then and. And I just understood that this is going to be the, the path of my life. So I never, I never went to university to, to do any, any kind of degrees. But part of my professional life, I got a lot of experience in a lot of tools that allowed me to, to conduct very, very advanced research.
So I'm very hands on. I think I'm, I'm calling myself developer data scientist maybe. I dunno. I, I did a lot in the last 12 years. I did some c CPP programming and and algorithms and modeling and autonomous vehicles and algo trading and cloud management and and different kind of stuff. And all of them, you know, I choose every position in my life in order to become.
The person I need to be in order to open a company. Like I had a vision that I want to do something and I know that I need to, to understand a big percentage of the stack in order to be able to know what actually happening. Maybe I'm free control in a way. So I choose very specifically and in in attention spec specific job that will, that will make me to gain those, those tools by the way.
Like, and, and this is my way to, to learn. Like if I want to study something new, I'm finding a job in, in this field.
And how do you find that job that you still don't know much about it
yet? I don't know. I, I think I'm have something, something compelling and convincing in, in job I. I'm not sure why people believe in me.
I never understood it, but I'm constantly getting jobs without any background in specific field. I, I got my first job as CPP programming for very specific and very complicated evolutionary algorithms for detecting and tracking after thousands of, of sensors without writing one line in cpp. But they just convinced them that they can learn and they learned in two weeks and they did.
And they did well. It worked very well. And also in the algo trading afterwards. And the autonomous vehicle never did near neural network before. Well, not for vision. I did some neural networks around 2014 for specific data. But yeah, you just get in there and people have face, it's very interesting.
It's very, it's very different. Like you cannot, you cannot predict it. Right. You just trust and it's happening. Yeah.
And how do you know, do you learn these new, these new fields for, for example, just, I know that a lot of people because of chat, G P T and other cool models want to trans transition into artificial intelligence and you've been learning by your own.
So I assume most certainly with online resources or books or something. Would you have anything you'd recommend for someone to get into the field from a different background?
Well, I, I got into the field intentionally and I devoted myself to, to artificial intelligence around intelligence around 2014.
And back then you had maybe, I dunno, five to 10 courses online in course and ude me and I did them all watched all the videos that had information in YouTube and read anything. And very quickly I understood that reading a paper. It's amazing. It's a, it's an amazing method that he had for a few years and habit to make a coffee and read one paper at least every day.
I would wake up and I got to times that I could finish on, understand the paper in, in 20, 30 minutes, maybe 40 minutes if it was very long one. But every day I would, I would read a paper, I make myself a list, and it's, it's amazing. And then, and then it's nice, you know, people, not just people writing papers exactly for that.
And it works like papers is amazing. And try when you have a work that requiring from you to know stuff in order to succeed, your understanding of of the material is different. Not only because you, you're going to use it also because, because you have a need to understand, and then the, the understanding is, is coming with pleasure because you have a need for it.
So if you want to study something to find a job, I think it's, it's much, it's much effective. Then go to the, you know, academy maybe. Mm-hmm. Now, when, when we developed when I'm developing a certain product, and I understand that in order to do that well I need to understand in magnetic field, I have a lot incentive, a lot of incentive to, to do whatever I can when I'm managing to understand something, it's not just for the sake of understanding I'm, I'm progressing towards a target.
Yeah. So you would re recommend to have some kind of incentive to learn something like the, the additional stress will, will help you better understand and process information,
I assume. Yeah, like find a reason that you want to study it. Make it tangible as you can. Don't just, you know, say I want to cure cancer.
It's important to cure cancer. And start to, to study. Make it tangible like, like your study and the effort you invest in, gonna make a difference in, in something that you care about. And then, and then when you'll study, you also will have different kind of energy, different kind of motive towards it, but you also will, right when you, when you get there, when you'll get a new understanding, when you manage to figure something out, it'll have much more meaning behind this, you know, pleasure of understanding stuff.
Yeah, I think that for me it's very, very important. I'm not sure I would be able to succeed in, in university. I'm not sure that I'm this kind of material, but I could write really great papers when I was 16 and, and I could solve very complex issues. I did a lot of, I did a lot of technology for, for the last 14 years and, and a very complicated one.
And still, I'm not sure that I would end the university. It's not, not sure I have this type
of brain. The regular university is definitely different and not, it's, it's unfortunate because a lot of people are in your situation as well where the classical path with collegian University isn't suited to them, but they, they basically have to do it and you are demonstrating that you don't have to do it.
But you may be a, a special case with a very high intelligence. So that may not be to anyone.
I'm not sure I'm a special case. I think that I'm a very lucky case. I'm truly think that, I'm not sure that I'm truly think that I won't be able to pass university well, as many people are. But I am a good developer, so I'm just wondering how many people that couldn't pass university and are possibly good developers just miss their opportunity because of expectations of, of society.
Yeah. Right. And it, it sucks or we have a great people around us that, that could achieve great stuff, but just because they're not fitting this way of thinking, those academic organizations are expecting everyone to think it just killed their, their opportunities. I think that I just had a lot of luck.
Like if we, if we'll move and, and find all the very specific events that happened in my life that allowed me to start working it very early work in very important project in the Israeli government achieve An experience in different, in different startups in my lifetime without a degree, and, and opening this startup 10 lip, like the chances is very, very low.
You can, you can understand that it's, it's all about throwing the dice properly. There is, it's, it's much of it. And then maybe I may be a bit more
trying more than more, most of the people I'm pushing really hard. I'm not resting. I'm working, think in average age and the last 12 years, I worked more than 12 hours a day.
And it's mostly because of the motivation where you have a goal and you, you basically need to learn and to, to produce something.
Just it, it is just like, I, I very understand what you are saying just because I do the same thing, but with. Due YouTube videos, basically where I tried to force myself to learn more efficiently by doing a video around a specific topic, which helped me a lot during my master's, just because my master's was very similar to a PhD.
And so it was at my own pace, and I, I just wasn't able to, to motivate myself to learning and reading papers unless I could do something out of it. And so I, I started doing blogs and YouTube videos just to force myself to read those papers and understand them and then be able to, to have some kind of output through that.
And so that, I think that's something that is very, I don't know, not precious, but very important for when learning something new. I, I think lots of people, for example, the ones that are transitioning into AI should try to, Have a goal in mind and then learn rather than just doing courses until, like, as you said, back in the days there, there were maybe 10 courses online, but now there are thousands.
So you can do courses indefinitely and, and never really enter the field. And that's, that's one of the, the danger that some people get into. And so I think aiming for something applied. For example, just when I, I I wanted to learn how to, how to code a mobile app. And so I just brainstormed with some friends on what kind of app we could build.
And once I had an id, I, I learned by building the app, which I believe is much more effective than just trying to learn
something and it makes sense, then just makes sense to study. Cause no, why, why you doing it for me? Yeah. To, to, to act from the right places. It's, it's hard that everyone need to get better in like, to find the reason you're doing stuff.
Yeah, so, so I, I think that I'm calling myself a data scientist for that 12 less years. I very hands on. And now, now I'm also startup co-founder, which is interesting experience. It's different.
It's hard. Yes. You mentioned the startup is Tenor Leap. So maybe we can dive into the, the main topic of this interview, which is explainability ai, but we will come back to that first.
Could you maybe just give us a little introduction of what, what is Tensor Leap and, and what do you do there?
Sure. So tensor Leap is, we call it applied explainability platform. It's a tool for. For developers, for, for data scientists that working on artificial int intelligence models. The motive to open the company were, we, we looked in the world and we saw, you know, even in 2000 when we did it 1918, we saw how how people developing newer networks and so many parts in critical parts of the development paradigm were relied on guesswork and anxious.
And it just disrupted, it just took out all the signs from a very scientific process. The way we constructed data sets. We just collecting randomly samples the way we, we testing it. By lack, if you are tested, is representing something in production, some, you know, some distribution of information to production, you might be able to.
Guess what will be the results in total. But so many, so many staff rely on, on guesswork. We try to understand why is it and what happened in, in this process. We understood that the lack of explainability, it's, it's a root cause for many, many different parts in this development paradigm. The idea that that developer using guesswork and, and Ansys in order to progress with their artificial int intelligence project.
You know, 12 years ago, I, I worked on, on the Magnetic Field project and we simulated the, the magnetic field completely. I studied books of physics and studied about materials. I studied about everything and, and the simulation of reality were perfect, very unstable because, you know, we cannot. Model reality perfectly.
It's very hard. But it, it was deterministic model, very complex, very huge, huge deterministic models. And then we started to do a bit statistics afterwards in order to round some corner to try to get to a bit more robustness. So you losing a, you are losing a bit information, you get, you become a bit more statistics, but still in this, in this distance between how detailed and specific you are to how flexible and, and robust you are, you're trying to find a balance.
And then we move from statistics to machine learning and we try to, you know, we said, okay, so we want, we wanna try all those rules about when it'll behave in this way or other. We'll give it the basic features that we decided are important and then we'll we'll open this machine learning will know how to balance those feature well.
And then, and then we did neural networks, we did deep learning and, and we said right to, to this process of feature extraction. It's way too complex, so I want the model to do it for myself. I'm just gonna describe my model some general idea of how the, how, how the dimension, how the domain looks like on which dimensions the information is, is distributing, and which way we can extract the features when the model will extract the features by itself.
And slowly, we, since, you know, 15 years ago we used to write, most of the people write very complex deterministic models to statistics, to machine learning, to to newer network. We, we stepped out of it and slowly we got less familiar with the domain. Mm-hmm. If today I need to do the project about magnetic fields from 12 years ago, I want to understand it as well, say I did back then because I won't need to, I just will, yeah.
Write some algorithm that will predict and learn where is the magnetic film will do whatever we'll do, I don't care. Right. But what happened there is that we do not understand the domain we, we working on. We do not understand how those neural network works. And so when it failed, you're starting to guess.
And when you're trying to, to teach or to train some model, you need to, you need to understand something about, you know how to construct these data sets, what kind of, of scenarios, what kind of edge cases population you have. And, and you're starting to guess a lot. I think that somewhere in this, in this process, from the journalistic to statistics to ml to deep planning, we just needed to stop somewhere and say, okay, now we need a different development product.
We constantly, like when we did random Forest and NG Boost and all of those models, you could train in two hours thousandths models, and you're doing it and it's fine, and you'll take the best one and maybe it works, right? And when you get results, you can just query some s SQL and, and find most of the edge cases because your table of features, you don't have thousands and thousands of pixels with some relations in a video.
You, you can find those you can find those edge cases with, with simple queries. But the move from structure data to unstructured data, from tables of information to videos, text, genetic data point cloud, all those unstructured information, just changing so much of the, of the game training newer network takes weeks for part of my, of our clients.
They cannot train thousands of neural networks and then take the best one. Mm-hmm. So, so 10 is here in order to develop an event find new explainability techniques and integrate them within the development process of neural networks.
And could you give a very short and clear explanation of what, what is ex explainability in ai?
And you mentioned you can give basically practical techniques or real techniques, applied techniques. Could you give an example of one one explainability AI technique? For the people listening that may not be familiar with explainability AI or xai,
we can say that there is too kind. People can mean to two different things when they're speaking about explainability.
You have explainability for the product itself. Let's say that you develop some newer network and integrating it into a product, and you need to explain your clients why you getting decisions, those explanations, people expecting to get something in a very human level. Yeah. Explanations. It's a bit harder.
It's a bit, we, we are not there yet. The science, but I, I believe that we'll gather. And the other explainability, which is the one that we are, we are more active in is just explainability that applies some mathematical techniques on your network. Most of the techniques are deterministic and providing some visual assets that will allow us to.
To understand the, the way of thinking of this snow and which feature feature they, they used. So for example, if we'll take a neural network that perceives images, for example, in searching cats and docs, we'll be able to take, to apply some mathematical operations on this model, while is predicting one specific sample in order to understand which features in the image cause the model to take a decision.
Right? So let's say that we, inferring an image will start to, we have many neurons. Each one of them learn some different features, which mean that every neuron creating a bit more different projection of the sample on, on some feature maps. And in each layer we'll get a new representations of of the same input.
Some of those representations can be used and more correlated with certain kind of prediction of the newer network. So for example, we'll take. The, the layering, the input and will create the root for certain neurons. For example, those that represent dog towards some layer in the model will be able to calculate the gradient between the neuron that critical the dog to all the neurons in the layer, and then will be able to understand that this specific neuron influencing the model a lot in order to get to guess that this dog and not a cat.
And then by tracing back the activation and fa finding where this feature actually were localized in, we can understand which area in the image caused this specific filter to fire and the model to take certain prediction. And there is, there is a few kind of explainability, let's split it to two.
There is local explainability and global explainability where local explainability is exactly that. It's trying to explain why a model took a decision on one sample and a global explainability is. In general on all dataset. So for example, potentially what we are doing, we're tracking after all the activation in those neural networks, we, we are calculating for each activation energy and entropy.
Some of them calculating mutual information between the prediction between different clusters in the model itself, which allow us to understand which features learn the most informative information, and then we construct in some latent space we just extracting activations from different fields in the model.
We're building some vector database with all the data sets and all the most important features index there. And then we'll be able to, and then we are able to search for different clusters and different populations. So, you know, if this process of the model of extracting features. We can rely on the idea that, you know, if it's guessed well, it means that he managed to study some features.
And if we can rely on the idea that it studied legit features or some of them, we can use those features in order to define different scenarios in the dataset. So for example, if we have thousand samples that fire in on exactly the same features, those thousand samples look alike. Cause they have the same features, right?
And if I have eyes like yours, I eyebrows like yours, hair like yours, we really look like you. So so this is what we are doing. We, we collecting all this information on the, all the neural networks features, and then we are projecting all the data set on the most important features in the model itself.
And then we searching with unsupervised techniques, different population, and then we trying to characterize those population, characterize the, trying to find the. The clusters, the, the features that express the most are very high information, again, with the input, which mean that those population probably tending to overfitting or we searching for populations that has low representation in the training set.
And then we can recommend the clients, Hey, bring us some unleveled data and we'll tell you which data it's better for you to label right now. Right. So we we're trying to find a lot of populations, and this is kind of global explainability.
So explainability would be used, well, should be used by anyone training or fine tuning or working with AI models.
And I assume it's mainly used to improve the results, but also to understand them or like improve the results by understanding them. And so I wonder if you could maybe, Give a very specific example. No worries if not, but like on the fly, for example, for the recent SAM model, which was a segment anything model, it's basically just a model for doing segmentation.
Could you think of one explainability technique that could be used to improve or better understand the results on this specific application?
So, you know, all those models are working the same in the terms that they are feature search models. They're all searching features that they can use in order to perform certain task, which kind of task can be analyzed different.
For example, if you are, if you're running on. If you're doing classification and you want to understand the way the model took decisions, you need to understand the decoder parts. And then you know how to run the ves. For example, you know how to create derivatives from the prediction neurons that symbolizing something to hold some latent based in the model.
And, and if you do an object detection, you can do it in a different different resolution, and you can speak on, you can create a, from each one of the neurons that represent some kind of instance in the image, right? A B box, a specific B box. And in each one of those algorithms, you'll have a different decoder.
And by understanding the decoder, you can write a very specific kind of explainability techniques. And it's all the idea, everything in neural networks is a, it's a, it's a process that's searching features from some unstructured, unstructured dimension. Sharing features, generalizing features, projecting the data on, on a lot of representations, more abstract, more simple to analyze, and then decoding them to, to a certain shape or decoding them into a certain certain representation that we can just, you know, apply some deterministic code after and take decisions.
And this some algorithm, it also has a very specific kind of, of decoder and there is different ways to tackle this decoder. You can understand that each pixel, it's an instance, and from each pixel you can create a derivatives because it's instance semantic segmentation techniques. You can create derivatives not from a pixel, but from the old instance itself, and then understand what caused for an instance to appear.
And you'll get different ideas. We, we did play with it a bit and because the model is not small many, if you, if you are taking the latest layer, many of the hit maps you'll receive will be huge and it'll be hard for you to understand. So what we did, which just keep going backward, and we just developing an techniques now that constantly going backward in the model, more and more shallow, creating more and more derivatives, recursively in order to understand, okay, so this, the neurons that in the end of the network was sensitive to, to information somewhere here.
And this neuron were sensitive to information a bit more specific. And then we going back and understanding which feature are constructed this very general feature, right? So we'll get imap within imap within pump, within an imap that explaining why specifically this instance was created.
And you mentioned it is basically very similar from one application to another, where it's a is it's applied, of course it, it'll be adapted to the application, but it's in short, similar and using the same technique.
And I assume, is it the same for the different architectures as well? For example, if we are doing image classification and we either use a convolution neural network or a vit, a transformer based vision network, would, would, would they be the same explainability technique? But just the computation will be a bit different just because of the math, but the visualization produced and everything, the same technique will be applied.
There is a ways to, to generalize those woos, and you must do that. The idea is that it's right when you take an image, the order of the pixels are very important and therefore people using convolutions because it able to find patterns from in such an old, in such as all the information. And in other cases you, you'll use LSTM in order to generalize the information.
Different, an old idea in your data. The data distribute in certain way and you trying to come up with mathematical operations that can. Be sensitive to, to generalization of this information. You know, so the first convolution they made, it's basically sharing the weights from different parts in the image.
It's sharing their understanding. It managed to learn a pattern and it's searching it in different places. And when they did transformers, they, they wanted to share and to be able to share information from non concise, non, non closed areas in the image. And the idea is it's interesting and you do need to understand it.
So the way we, when we working on neural networks, we taking the models in OnX, for example, and we passing it all and we completely understand each one of the pop of the operation. We understand on which dimension it's, it's, it's creating, it's trying to generalize information and when you want to calculate, for example Mutual information or, or entropy of certain prediction.
You have to understand the dimensions of, of the output of those operations. You need to understand on which dimension it, you try to generalize the information. And if you are running on lstm, you do need to understand the state. And if you are running on transformers, you need to understand where you have the channels.
So once you understand on which dimension and in which way the operation generalized the information you, you can generalize some process that will bit to apply the similar grid in each one of them. Sometimes you need to normalize different values because different operation tending to give you values in different scales, but you know it's technicalities.
Yeah. And when you understand that each one of them is, is a feature and the feature is something that's sensitive to pattern or to some, Complex pattern of, of few patterns that working together. And you understand that you can, that it's a model within a model, within a model, within a model that's sensitive to all this information, you can apply almost the same techniques.
Just need to understand are you measuring each one of the features? Why? If you are working, for example, in VI and you have also convolution models and also a transformer mechanism, you need to understand what is the patches work. And, you know, in order to be able, how to measure and to understand that this transformer feature are important as this convolutional operation and pattern.
And it's just need to, to trick a bit the, the techniques. But after you did that and you managed to extract and to represent it in some way, in a latent space, you can use this latent space as to just you know, set of features that you manage to extract from your model, from your data. And then it's, it's, it's all the same from there.
So, so yes, you can generalize and you can apply almost the same techniques on any kind of neural network. There is some technicalities, and that will require from us to understand exactly how the mathematical operation working, how you can reduce the information without damaging the information you're trying to represent with in certain light spaces.
And can we do anything when we work with pre-trained models, for example, we, a lot of people right now, just the very basic example is again, image classification. And instead of, of taking, for example, a cnn, a commercial neural network and training it from scratch on our data set, we will first just download an ImageNet trained model and then fine tune or retrain or do whatever on our data to make it perform better.
But then you don't have access to the whole training process. So is there a way to use explainability when the, when you haven't trained the model, just when you, you already have it well trained and very powerful. Like, is there something you can do to better understand the results if the, the, the model is already available?
Yeah, so, well, first of all, we need to, we need a better reason to do explainability. If you're trying to explain a specific prediction in productions, of course you'll be able to take the model and to find the features that took part in this specific prediction, this specific sample, and displayed outputs.
And then to get some hints about where the information that the model is when you took a certain prediction, you'll be able to extract those features from all the samples in production at the same moment and search. Places that the model took decisions from the same features. It's also very interesting, right?
If I'll tell you, it thought that this is a dog. And on this one with images, it thought that it's a dog from the same reason, from the same features. And this is the differences between them. So it's all, it's all you know, a game of how, how you are, you're playing with those features. You don't need, you don't have to train your model on, on your data in order to do so.
But when you do train your model in data, those techniques can have a very strong influence on the development process, right? Because if I can tell you now you have 2 million images that looks exactly the same from this population and 500 images from this population, you're starting to understand how the features expressed in your dataset and how the information is distributing your dataset.
You can get to much better dataset. We just don't know and we can't know. You see, like if we could keep writing theistic algorithms, we would like, you can test it. I, I can write test for theistic algorithm. It's amazing. But the world is too complex and people are understood that they cannot handle the complexity in autonomous speaker.
It's too big. Like we will never be able to, to define and to represent all the complexity, all the patterns that an image can see. So, so we understood that and, and we went to a process that's searching features. So by definition, everywhere you use neural networks, it's a very complex process that you cannot, you would not think about all the features.
So if you wanna think about all the features, how can you make sure that you're testing it properly in the right places? How you can make sure that you have the right samples in the dataset, right? So there is, there is. Trapped over there. And and I think that it's a combination of both of them.
Like you need to constantly apply explainability techniques on your model and by that to learn more and more about the domain activate and then to create a better processes that understand how the information distributing constantly cur your data set and constantly find more and more edge cases for that you, you just need to understand that you need to track after them cause they tending to fail cause of complexity or ambiguity or anything.
And how would you recommend someone, for example, a student that is working with any kind of model how and doesn't know about explainability techniques, how would you recommend this person to get into this explainability field and just, Use it to better understand their model and improve their model, like the basics to know when you want to use these techniques to improve your model and you need to better understand the results, where can you start doing that and what do you need to know?
Yeah. Well, everyone can use 10, 10 lip. It's full of explainability tools for that. I think that there is a bunch of great papers in the field that come into to push and help explainability to emerge properly and to provide people the tools they need in order to, to understand the model, to gain confidence.
You know, now when you're trying to. Move all these procedures for a new autonomous vehicle or, or to, to get an FDA stamp, you will, you have to provide external ability with it. So there is a lot of work in those fields. I'm not aware of many tools that, you know, very mature that, that exposing explainability for the development process itself.
Probably there is a bunch of them. I think that it's more change of mind. You know, for four years ago, neural network in a black box, it was, it was obvious, right? People have claimed, and there is nothing to do. It's too big. We won't be able to explain it, and it's not the case anymore. Mm-hmm. It's mostly just people need to embrace and to understand the idea that we need.
We can, and we should invest some effort in explaining those models. It's hard, but it must, we cannot release autonomous vehicle to destroy without understanding how they works. And if we tested them properly, what kind of scenarios they will be sensitive to. It's impossible. And I think that, I think that people just need to get used to the idea that it's, it's possible to explain them now that we have the amount of computation we can conduct and a bit more advanced techniques to research those algorithms.
But, you know, well guys read about class activation marks about embedding spaces about similarities of of spaces. And should
there be a specific team dedicated to understanding and explaining the models that is separate from the, the people that are developing those models. Or should everyone creating artificial intelligence models, Also be familiar with explainability and be able to understand the decision of the models they create.
It's a very nice idea to have in a team, in a development team, a team that in charge on explainability and team, that in charge on, on modeling itself. You like if you were trying to optimize the focus of the personas? Yeah, I think that explainability is a domain expertise that can be applied on different domains.
So I can be a, a professional and explainability and they can do explainability to hand with, of models without drilling down into the domain itself, not necessarily so professionally. You know, people did fronted backend DevOps distributed this world. I do believe that. When you working with explainability, you're gaining so much about how the model is working, what kind of features it's tending to land, how the, how the reality is distributing.
And I think that no matter how much 10 will, will do, great intuition will be a very strong part in, in data scientist because the amount of choices you can, you need to make in a day. It's, it's enormous. But I think that accessibility can help a lot in building those intuitions. I do believe that we manage to reduce the, the guesswork they do in, in 90% day, like doing in much less guesses.
But it's also, it's still important to build an intuition when you're working on, on a certain domain. So I think that it's very healthy for any kind of data scientist that's working on artificial intelligence. To apply explainability on his Zoom model. But it's very interesting in a big corporation, I can see it happening that, you know, in a few years there will have explainability teams that will charge on apply explainability techniques on, on the models that the company's building.
And do you think right, right now you mentioned that we are not yet at a level of, of being able to give like human easily understandable explanations from the model that, that any human can understand. And so the, the main background of someone working with explainability sh should be some kind of data scientist, but also I assume for very specific cases you also need an, an expert in the field.
To tell you if the model is, is making the right decision or not. And like, if, if the model is explanation is right or not, you may need an expert in the, the specific field.
It's not an, it's not easy to trust those models, especially how they coming out today. And I still think that there is a few stuff we can do in order together and we should gather with a strong, strong understanding.
But in order to do that, we need to develop them a bit differently. There is some work that progressing pretty well with expanding models. There is even a paper, it's, it's really a great one, I think it's called the Mona, is a textual description of visual features in your own networks. So exactly they did with clip, they applied on feature maps within the model itself.
So it learned and it tells you it's a doc because of the Ponty here. He found pointy hears and it, he described those visual features within the model. So it's starting, it, it'll gather at some point, I believe so I'm not sure how fast it'll be, but I think that before we get in there we can easily understand, for example, that if a neural network getting decision based on certain features, we can be confident that those features are legit, maybe, right.
So maybe we can map the legit features in the model and and the relation between them. Maybe every time you get given a predictions for, I dunno pathologists can, for example, if you found a cancer or not, some of the prediction, you can estimate the amount of uncertainty the model has. So models tend to be very confident about the results.
It's a hundred percent cow when it's a car, but it's an hundred percent because it's usually happens because our data sets are. Very unbalanced and we very aggressive with our loss functions, so we tending to have a very confident models even when they land. Yeah. So, and, and it's an issue, but there is different ways to tackle this issue.
And I think that all those ways are great ways to give us confident about if the model now needs another professionalized to validate the prediction it has or, or not. So for example you can duplicate the prediction in the training phase to run some dropout. And then when you predict and you, you allegedly asking the model different models on the same prediction and then they averaging how many times each one of the prediction happens and then you getting some kind of confident or another models that estimating the error of the model.
You're training one model. And you're training another model, they're just estimating error. And then in production you can ask what is the pr is it a dog or a or cat? And then you're asking the other model, what is the chances that this model is, is, is wrong now the first model gave you a hundred percent, but another model can give you a bit more confident staff.
Some works that we are doing in 10. Now we constantly, you know, because we are very strongly into this slated space. So for example, if we found a very valid and strong population that we manage to characterize well and to understand well, and to find all the weighted information is distributed in real time, we can predict and understand if it's an example that is common in certain populations.
So for example, if we have a latent based and you have groups, we'll take a group. And if the sample fell in the middle, we more confident. About the demo, that it's a legit prediction cause it's something that you already so many times maybe. So there is different kind of games. You, you can run on it.
It's a bit more complex. You need to generalize it. Well, you need to understand how many features, how deep they are, how general they are in order to, to make it properly. For example, if you have some features that are very high information, again, with the input and you get in a cluster that emerge there and then a new samples that from production, you still need to have a low confident in the, in the results because his ability to generalize information is very low in this population.
Right? So you can normalize it by different techniques, but at center at, at some point on, I believe that most of the dataset you'll have in production also, you'll be able to feel much more confident and to provide testimonials that it works. So for example, what we can see in many places, I think that the FDA has already did it al already asking such as explainability techniques, give me, take a certain sample, make a prediction, and show me other prediction we made from the same reason.
Right? So it's, we will keep, keep progress and develop more and more techniques that will allow us to rely less and less on experts.
So that's, you exactly answered my, the question I had regarding using explainability. Both we, early, earlier in the discussion we talked about using explainability to improve your results and improve the results, basically.
And my question was about how to use it for the other side where the user or client just wants some explanation or. At least a little bit more details of why the model told you that this was a Doug, not very long ago, you just had, as you said, models with very high confidence on most, most decisions that they took.
For example, they, they could just say that in an image there was a dug and with like 95% certainty, whereas it isn't even a dug. It's just because there are, there, there's grass in the image and the model is just used to when, when there's grass, there's a dug. So like, it, it's confident, but it's confident in failing and so.
It's really nice to, to see more explainability techniques being used to better explain the, the results to the users and not just to the, the people training the models. And speaking of which, I think it may not be possible to do that, but I wonder if there's a way or a specific technique that you can use to explain models that are used through APIs such as chat, G p T, that pretty much everyone uses right now.
Is there something that we can do to better understand their decision or, or why the answer the way they did?
Well, you know back then legit techniques in ML were in the models that doesn't have a will. Explainability when you cannot apply really well in explainability on certain model. Many people used to train another model that it's easy to explain and assume that the features they will search will be similar.
I think that when you work in with an api L LM models in a, with an api, there is still a bunch you can do and should it, it's a, it's huge problem today. It's so easy to come up with a super cool and advanced and strong technology with all those tools, but it's gonna make us, it's gonna make a lot of issues because we won't be able, we are, we, we are giving up on testing, we're giving up on monitoring, giving up on, on so much, and they think that, We just need to, to tackle it with the understanding that it's, it's an api.
They're getting unstructured data and, and risk and receiving unstructured data from the other end. So maybe, maybe it's fine to, to index the, to extra extract the features. Let's take be for example, and when you're asking this API, extract the features before when you get in response, extract the feature again, index them to some vector database, have some similarity understanding, and then you can, for example, monitor when you ask in the same questions in different words, how different is the answer in the unlabeled structure?
Right on, on your on your vector database, on your latent space, how different the answers are. You can assume that you want to keep on. Casual and well distributed answers. And, and maybe some more games like that. But all of those are not necessarily explainability techniques because they are not using the model that gave you the prediction.
Yeah. Which is, which is gonna be difficult. What they will do. They maybe one day we'll, you know, extract another API that monitoring the model itself and providing them explainability in real time for certain predictions. But those models are huge and so complex. Yeah. Yeah. It'll take us time together.
Maybe
four years. Eh, it's really funny because back in the days, well, it's not even long, a long time ago, but I always say that it's, it, it just goes so fast. But like we, we used to say that we will need, ironically, that we will need deep nets to understand deep nets. And right now it's like, Pretty much a norm.
And you are, you are explaining that using a model on another model's results in a way that this, this is something very simple and straightforward and that like we can easily do. It's, it's very funny to me compared to where we were a few years ago, where just doing a deep networks, the deep network was something impossible or very complicated, and now we can just use one to better understand another one.
It's, it's pretty cool, pretty funny. And so I assume this is also the ultimate goal of explainability AI is to basically no longer have a black box anymore, is to do anything we can to understand the model we have, right?
Yeah, I think so. I think that and, and the impact has, has to happen you know, in the, in the development chain because.
You know, today when we deploying a new model to production and we are running it on our test set and getting 97% accuracy, which is a very high number that we saw, that'll be good if we get to just because it's it's an issue. And those hundred, 500,000 samples data sets, test sets are, you know, many time oriented and by as to five big populations.
So you have five really common population that you're predicting very well on, and hundreds that you're failing without mind. And they can, you know, represent a very real scenarios in reality that we just will never know that we are failing every time we deploy a new model. And explainability, I think, is the only way that will allow us to.
Work with future searching algorithms like neural networks and make sure that we are not failing it on, on new ones. We'll never be God, we'll never understand completely the reality. We'll never be able to do perfect algorithm from work on all the scenarios that exist and will be exist in the futures.
But we do need to apply the same amount of effort and complexity in testing and constructing those models as they are. Right. Because we, to get 97% accuracy on one test set, it's, it's not, it's not proving me anything and I don't think that you're proving anyone anything. Yeah. So, so truly half that explainability will allow us to improve dramatically the way we, we testing those models and the confidence of the world for those technologies and, you know,
And do you think it'll also allow us to better understand our own brain?
I feel like, I don't know how much they are linked. Well, not they are, like, initially they were linked, but now I doubt neural networks, artificial neural networks work the same way that our neural network works. But do you think, well first are, are you taking some like cognitive related approaches and use it and try to use them on artificial networks?
Did you, did you search into the neurosciences and, and try to implement some of their IDs or, or vice versa?
Yeah, yeah, definitely. Well, not vice versa, only from there two hours because I never got to, to work on biological. Neural networks, but maybe one day. I have one data scientist that doing the research about the similarities between our artificial neural networks and, you know, theological neural networks are the operating similarities.
And we did talk some nice ideas from there. For example, when you take a group of group of patients and you're trying to understand how their brain working differently, so you're creating some kind of self-expressed metrics that giving you some kind of signature of how one brain works on a group of samples.
And we apply the same techniques with intensely in order to be able to compare to different models. And it works great. So we do have some, we do took from, from neuroscience, some some cool techniques that they used when they researching on biological brains and And I think that there is similarities.
It works almost the same. And there is, you know, because of the complexity and, and the structure, lot of differences, but it still operate almost the same. It'll take us a while, I think maybe 20 years to be able to learn something about our brain from artificial intelligent research. Only because the brains that we working on today are so simple relative to the human brain.
You know, we'll get, we might get there in 20 years but I think that we are not that close yet. But also, I'm not huge experts in your science, Monique.
It's definitely explainability in, in artificial intelligence is definitely a very good field to be in. If you, if we want to better understand our own brain as well, just in the, in the, well, I don't know how far future, but in the.
In the future how do you see the explainability AI field in five years? Will we be able to completely understand the models and, and build them like very optimally or will we just progress at the same speed the research progresses and developing new models so we still won't be able to understand them?
What will it be like? Well, I,
I believe that in five years we'll be in a different place towards explainability, I think will be much better. People starting to understand again, more and more how, how it's important to explainability and to understand what you're doing and why is stuff happening. Yeah, so I, I think that in five years we'll get to a much better techniques, much progress tools that will allow us to develop it much better, but much clearer.
I think we'll, we'll, it, it'll be commodity to understand your model and to provide expandability with it. The world in Ghana invest a lot of efforts those direction, and we already proved that when we deciding, you know, this community deciding that something is important, they, they achieving it. And I think that's relative to the gain the world will have from a well defined and and an advanced external ability techniques towards the amount of work we need to do towards this direction.
It, it's a no-brainer. So I believe that in five years, the external ability techniques will be much better than we have today. And I'm pretty sure it's gonna look change completely the way we look on, on artificial neural networks. Awesome.
That's, that's exciting then. And is there any risk of using explainability techniques?
For example, when we say that the prediction of a cat is confident, the, the model is confident by 90% or something, using another model to do that, is there any risk in using that? Like will it will it lead to being too confident in our models because we assume they're making the right decisions, and in fact, it may be just another bias in the other model.
Is there any risk of using such techniques or how to mitigate those risks?
My, my belief towards those, you know, kind of questions is that relative to the nothing most of the companies doing today, Everything they'll do will make it better. Right? Like many times we demonstrating the abilities of our platform and people asking us, yes, but don't you create more confirmational bias to your explainability account?
And then you're more biased with the data set constructing for that. But I, I never end up into this direction with, yeah, it's better to not do that. Like relative to the nothing we are doing. Right. It might create a bit more confirmational bias. It might, you might get confident in explainability techniques that you shouldn't get such confident and, but relative to what we are doing today, it's a huge improvement.
Yeah, that definitely makes sense. And well, my, my last question, I, the last question I usually ask is, what is the project you are working on? But I assume you are mainly working of tensor leap. But if you are doing anything else, feel free to to share it with the audience or else just maybe quickly resay, like, summarize once again, who and why should we use Tensor Leap or explain V Techniques?
Just yeah, who should we use Tensor Leap and why? Yeah, sure.
So well, no, I'm, I'm, we work in Inten is a huge product, huge platform. We have, there is no chance I left time to do anything else. We work in 13, 14 hours a day. So I'm, I don't have any other project who should use 10 Lip and why. It's people that work in data scientists that working on neural networks.
Training them, monitoring them, and maintaining them on, on advance and complex tasks. Right? When the group of people get into such a point that they need to maintain modeling, production, get confident in what they do, they, it's, those are the people that should use Sten Lip. Tenille is not only a Python library.
It's a huge platform in order to be able to track after a hundred of thousands, sometimes millions of samples and millions of neurons, and to track after all those activation into index them properly, it's a cloud-based solution just for the people that's watching us. It's a cloud-based solution based on Kubernetes, and it can be deployed everywhere, but it's an heavy solution.
It's not it's not sample that it's easy to construct inhouse. It required a lot of DevOps and, and software architectural techniques that we'll be able to detect and to maintain this complex solution. So we did a lot of it. We called, we maintaining three or four different databases that each one of them really good in indexing and searching specific kind of information like vector databases and big data databases and, you know different ones, about four of them.
And we indexing all this information and we applying very advanced techniques in it. So we did already a lot of work in the last three years and we got to a really cool product. I think that can definitely and transform the way companies working on your networks every day. So. Especially for people that working on neural network need to make sure that you will work properly in production, understand where those, those neural network might fail and improve the way data sets are constructed.
This is this, those are the teams that should use it.
Perfect. And I completely agree with the tool being being great and just the team in general as well. I, I definitely recommend looking into tensor leap and explainability in, in general. I think it's a very important topic. And speaking of which, we will also have a video introducing explainability AI on the channel, so, which we, we worked on together.
So I think it'll be, it'll be very cool. And yeah, that's, those were pretty much all my questions. If, if you listening to the episode will like something more in depth on explainability, maybe we can. Work on some kind of practical tutorial. So let us know if you have any questions or would like any more information related to explainability in artificial intelligence.
And thank you very much for your time. I, I really appreciate it. It's, it's a, a long time. It's hard to find this time for you, especially with working over 12 hours a day. So I, I really appreciate all the insights you provided and, and your time. Thank you very much.